Things that are called ML/AI that really aren’t
So many products promise to be ML/AI when they are just an impressive algorithm. But smart isn't the same as intelligent when it comes to the minds of machines.
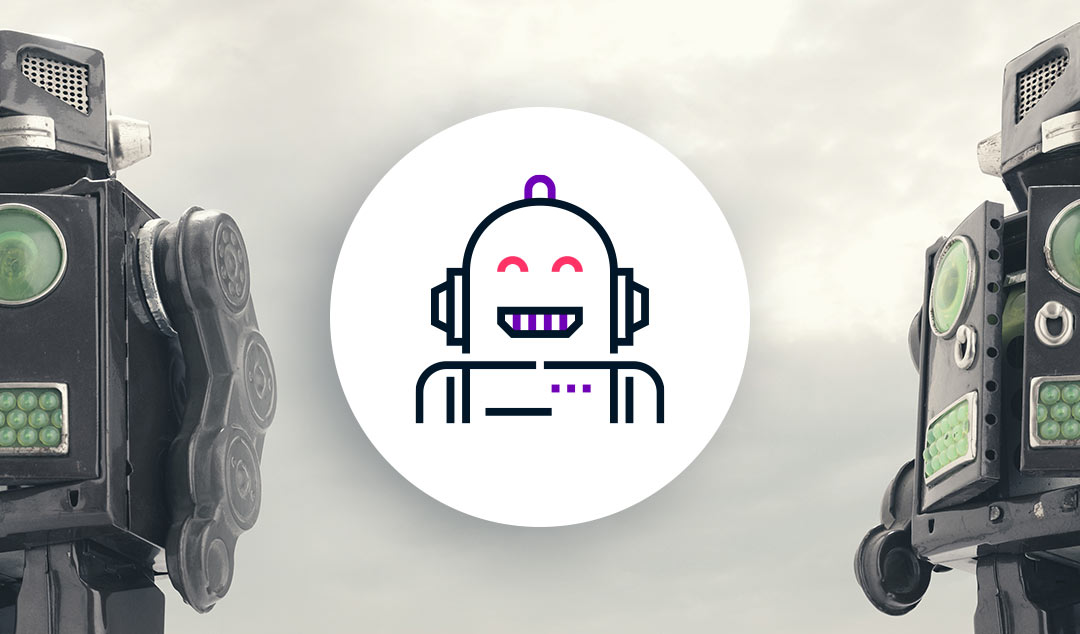
So many products promise to be machine learning or AI when they are just an impressive algorithm. But smart is not the same as intelligent when it comes to the minds of machines. Can you spot the difference?
Artificial Intelligence (AI) is a genuine technical term to describe something that doesn’t quite yet exist in a truly cognitive form. However, AI is also a marketing buzzword used to distinguish items with extra usability or computing-power oompfh. The acronym often attempts to differentiate ordinary things, such as phones, from extraordinary things of the same ilk, such smartphones.
Because there’s no legal governance over the use of AI in marketing, the label is abundantly applied to hardware or software use traditional algorithms as well as to things that actually learn. Calling all these things “smart” muddies the waters even more – and makes it difficult to make rational decisions.
“Many times companies use the term ‘artificial intelligence’ to describe technology that operates without human interaction, but most times it’s just a sophisticated algorithm,” says Scott George, CEO of U.S. Consumer Healthcare Advocacy Group (USCHAG), a consortium of healthcare professionals, institutions, and organizations. He cites website chatbots as an example, which some consider AI – but usually don’t meet the technical criteria.
“The confusion here is that for something to qualify as AI doesn’t actually require it to have an advanced form of cognition,” says Benjamin Nussbaum, AI/ML advisor to the Greystones Group, a technical support provider for the Department of Defense (DoD) and commercial clients. So many companies can tout what they do as AI is because the definition of AI was established back in the 1950s and only requires that a machine can do as well or better that which a human can do. “This opens the door for basic automation, analysis algorithms, etc. to all be categorized as AI,” adds Nussbaum.
Naturally, that is extremely confusing for anyone who wants to assess any system’s value. The average algorithm is so sophisticated today that spotting the difference can be nearly impossible for the average buyer.
The solution is to look at the system’s value without regard to how it’s built. If it genuinely uses AI or machine learning, great; but what matters is whether it makes life better.
“Ultimately, to the consumer, it doesn’t matter how the tech works as long as they get what they need and what is promised. For instance, we all use smartphones but would be hard pressed to explain the technology behind them,” says George.
“It’s the same with AI and ML. There are different permutations of each; however, deep machine learning is a differentiator in the space.” It’s important for investors to know the difference, George adds.
Investors are often stumped too, so venture capitalist firms and banks work to more clearly define products and the companies that make them. For example, a report by London-based venture capital firm MMC and Barclays Bank found that 2,830 startups in Europe were classified as being AI companies, but only 1,580 accurately fit the criteria.
The smart telltale signs
Much has been written to define AI and its subset machine learning (ML). But those technical explanations are little help to users, even if they could examine code or a system’s inner workings.
“ML is just using what has occurred in the past and statistics around it to guess when that will happen again,” Nussbaum explains. Many products claiming to use AI/ML do not employ any true form of cognition. “The best way to assess the AI/ML solution is to focus on which human tasks it will be able to do in a reliable manner and how it will interact with other humans on your team.”
There’s an easier rule of thumb to distinguish AI from ML and algorithms in general, suggests Rima Shah, link building analyst at Technostacks Infotech, an IT company based in India. “It’s more like ML is a bookworm and AI is practically-applied scientific database.”
In short, to sort science from marketing fiction, look at what the system needs, uses, and produces.
Things that are typically called AI/ML but are likely not
The easiest way to differentiate between sophisticated automation (laudatory in its own right) and ML and “smart” algorithms is whether the system requires instructions (as with automation) or if it does its thing with little input from its human overlords (ML, or at least built on ML).
Here are some things that are often called AI/ML but likely aren’t:
Coupon generators. They spit out coupons and offers based on your previous purchases. You see these recommendation engines at checkouts in drug stores and grocery stores. An algorithm predicts when you’re likely to run out of a previous purchase (such as dog food) and spits out a “right on time” coupon to entice you to buy it again. It does not know why you buy what you bought, and it doesn’t learn your preferences or behavior changes over time.
Online ad matching. You wonder why you’re seeing an ad on Facebook or elsewhere online for an item you just bought. This isn’t AI, because the algorithms typically analyze your search queries rather than your purchases. It doesn’t figure out why you’re searching for that item; it only notes your interest and spits out ads that match.
Chatbots. While ML and natural language search are hot trends in this space, most chatbots are automated FAQ responses pulled from a company’s knowledge base. In other words, the chatbot stores is a preprogrammed answer for every common question. But it’s merely a database lookup. Stray from the most-asked path and the chatbot goes mum.
Car diagnostics. This category is a bit tricky because a lot of AI/ML is used in today’s vehicles, with more to come. But for now, the application that shows you whether your car needs air in its tires or an oil change isn’t AI. It is the product of sophisticated algorithms that simply compare the current metrics with pre-established and programmed standards, e.g. oil should be changed every so many miles, and tires should contain x amount of air pressure.
The Internet of Things (IoT). Most IoT items are ordinary things outfitted with sensors and connected to the Internet. If there is any AI/ML in use, it’s usually in the cloud or on the database back end. Most typically, IoT applications collect and analyze user information, rather than to make the a consumer IoT device work better. For example, a smart home security camera may use traditional algorithms for reporting. If it uses ML/AI at all, it’s typically to analyze user behavior for sale to other data collectors, or to analyze activities in the local area to produce a larger crime study for law enforcement or insurance companies’ use.
An oversimplified way to determine if an application uses ML/AI is to note if it requires “training” and if so, how it’s trained. Cybersecurity vendors, for example, typically pre-train ML/AI to detect and preempt known cyberthreats. But ML cannot adapt to new threats without being deliberately trained for them. AI can, but it needs access to vast amounts of data on an ongoing basis in order to learn new threats on its own. Algorithms, even very advanced ones, simply do as they are told in their programming. The same is true of automation.
Ask vendors how the claimed AI/ML was trained and whether it requires ongoing “training” to function properly. Compare outputs for a final determination on whether performance is up to par with human performance in order to determine ultimate value regardless of what the software is using to do the job.
“In the end, it does not matter if a product has machine learning or not as long as the product accomplishes its intended goal. However, products should not make false promises as this points to an unreliable company,” says Dan Edmonson, CEO of Dronegenuity, a drone-based photography service.
Functionize’s software testing tools don’t claim to be artificial intelligence in the sense of predicting human behavior – but we are proud of the way we use it under-the-hood. We invite you to learn what we mean by intelligent performance software testing, and how it can deliver real insights.